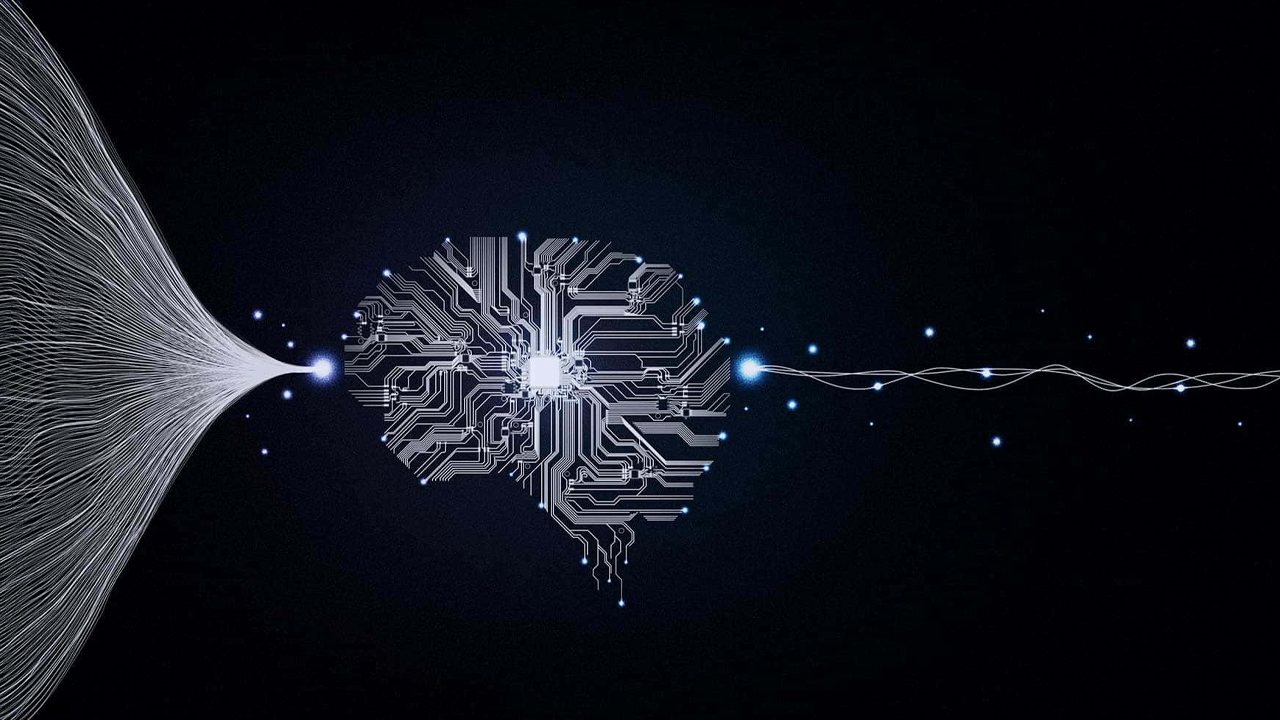
In today’s fast-paced monetary markets, automated buying and selling has come to be a cornerstone of current making an investment. Algorithms now execute a big element of worldwide trades, responding to marketplace conditions in milliseconds. But even as pace and efficiency are vital, threat management remains the holy grail of trading success. This is in which system getting to know (ML) steps in—reworking computerized trading from a rule-based totally technique right into a especially adaptive, chance-conscious machine.
The Evolution from Static Algorithms to Adaptive Intelligence
Traditional algorithmic buying and selling systems rely upon predefined regulations. For example, a machine may buy a stock while it drops five% and sell when it rises 10%. While powerful in certain conditions, those structures are brittle—they could fail while the marketplace behaves abruptly.
Machine mastering, alternatively, lets in systems to analyze from information, adapt to changing conditions, and perceive complex styles which might be invisible to static rule sets. Rather than being advised what to do, ML models are educated to recognize what a hit trades look like, and how danger elements evolve over time.
Core Ways Machine Learning Reduces Risk
- Predictive Analytics for Market Trends
ML models ingest great amounts of historical and real-time statistics—rate actions, trading volumes, economic signs, social sentiment, and greater—to forecast quick-term and lengthy-time period tendencies. This permits systems to proactively adjust their techniques primarily based on chance fashions, as opposed to reacting to marketplace movements when they arise. - Dynamic Risk Assessment
ML doesn’t simply optimize for returns—it may be taught to prioritize capital preservation. Models assess risk exposure in actual-time and alter positions as a consequence. For example, if a positive asset will become rather unstable, the version may lessen position size or growth hedge positions automatically. - Anomaly Detection
Machine getting to know is extremely good at identifying anomalies—unusual price moves, liquidity gaps, or suspicious buying and selling hobby. This allows investors keep away from flash crashes, manipulation activities, or algorithmic misfires, via routinely flagging or suspending trades below questionable situations. - Portfolio Optimization
Tools like the Inqubeta Trade Predictor are leveraging ML to assist investors no longer simplest discover promising change possibilities but additionally stability diversification, risk publicity, and expected returns. This level of precision could be impossible with manual strategies or inflexible algorithms. - Behavioral Finance Integration
ML models can analyze the behavior of market participants and integrate sentiment data (from information, social media, etc.) into their choice-making procedure. By accounting for human psychology—like worry, greed, or herd behavior—trading structures end up more robust and less at risk of being stuck off-shield with the aid of irrational marketplace moves.
Real-World Applications
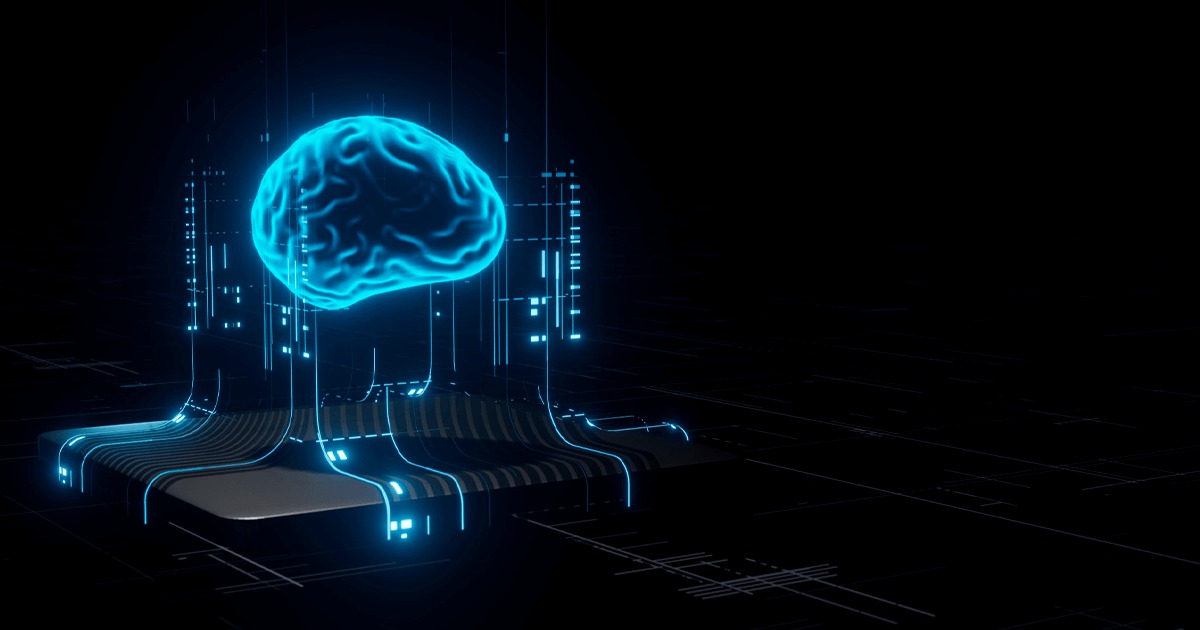
Large hedge funds and trading companies had been the usage of ML-pushed systems for years, but thanks to structures like the Inqubeta Trade Predictor, this technology is becoming reachable to retail investors as nicely. These structures provide users with AI-assisted change guidelines, actual-time analytics, and integrated hazard tests, giving everyday investors access to equipment that were once the domain of elite establishments.
Challenges and Considerations
While the blessings are significant, it is critical to note that machine gaining knowledge of isn't always infallible. Models are only as exact as the statistics they may be skilled on. Overfitting, terrible statistics nice, and lack of transparency in selection-making ("black box" conduct) are actual worries. That’s why present day ML platforms combine explainability capabilities, rigorous testing, and human oversight to ensure reliability and duty.
The Future of Risk-Aware Trading
As economic markets grow extra complicated and interconnected, device learning will maintain to adapt. Future trading systems will probable encompass multi-agent AI frameworks, deeper integration with macroeconomic modeling, and more state-of-the-art behavioral insights. The last intention? A trading surroundings where machines do not just execute trades—they control risk proactively and intelligently, making sure safer consequences for all members.
In the arena of automatic trading, velocity is vital—but survivability is everything. Machine mastering is proving to be a sport-changer by means of minimizing exposure to market shocks, optimizing techniques in actual-time, and making threat control smarter and extra adaptive. Platforms like Inqubeta Trade Predictor are at the vanguard of this variation, democratizing get entry to to modern-day chance-discount tools for traders of all stages.
Whether you are a beginner investor or an experienced quant, embracing ML-driven gear might be the smartest flow you make in navigating the markets of tomorrow.
AI trading platform GPT Trading trading technology Artificial Intelligence AI in Business AI in Finance AI in Healthcare Quantum Computing AI Automation AI Trading Algorithmic Trading Online Trading Quantum Finance